As the novel coronavirus that causes COVID-19 spreads across the globe, innovative thinking is needed more than ever to counter the devastating effects on the physical and socioeconomic health of individuals and communities. Innovations in healthcare delivery not yet fully realized prior to the pandemic, such as the adoption of telehealth, are moving to the mainstream. Artificial intelligence (AI) and machine learning are other important tools being adopted to manage and process massive amounts of data on the virus.
One specific application of AI during COVID-19 has been an attempt to use it to diagnose the disease with computed tomography (CT) imaging. Although the accuracy of AI in this setting is still being explored, as discussed in correspondence to the Lancet Digital Health on May 1, 2020, and in a blog from the Brookings Institute, the interest in, and research on, using AI in medical imaging during the pandemic may accelerate its use for medical imaging in general.1,2
Berend Stoel, PhD, a computer scientist in the division of medical image processing, Radiology, at Leiden University Medical Center, The Netherlands, thinks so. “There are already several research projects ongoing to apply AI in interpreting chest CTs of patients suspected of having COVID-19,” says Dr. Stoel, who published a review of current and potential uses of AI and machine learning in imaging in rheumatology in the January 2020 issue of RMD Open.3
“This will boost the AI development in imaging in general, and rheumatologic applications of AI will benefit from this as well,” Dr. Stoel says.
Along with imaging, AI and machine learning are being looked at for an array of potential applications within rheumatology, such as disease detection and stratification, prediction of disease flares, prediction of disease progression and use of genetic biomarkers to personalize treatment, as discussed in a 2020 review by Hügle et al.4
This is just a sampling of potential applications opening up for rheumatologists as physicians grapple with how to harness the power of big data and sophisticated technological processing tools.
Given the broad scope of research on AI and machine learning, this article focuses on the area in which these tools may first be applied in rheumatology practices—imaging.
“I think imaging is the entry for AI in rheumatology,” says Thomas Hügle, MD, PhD, head of, and professor in, the Department of Rheumatology, University Hospital, Lausanne, Switzerland. Crucial among the reasons for this is the wide availability of data in radiology on which AI and machine learning depends to create algorithms.
“Imaging is data driven, and data are what we really need for machine learning,” he says.
Use in Imaging
Dr. Hügle lists a number of benefits and examples of AI and machine learning in imaging for rheumatology. AI can:
- Assist radiologists and rheumatologists in improving efficient, accurate diagnosis. Example: AI analysis of musculoskeletal diseases is relatively easy given the binary questions asked (e.g., Are osteophytes present or not? Is joint space narrowing present or not? Is there calcification in the meniscus or not?). This is the type of repetitive task that provides an inroad to AI and machine learning;
- Generate automated reports, reducing the time clinicians spend on dictating radiologic reports, such as dual-energy X-ray absorptiometry findings, and providing more time for difficult cases or interacting with patients;
- Reduce the number of images needed for patients by automatically detecting and identifying features in images taken for other purposes. Example: An abdominal CT scan performed for other reasons may automatically provide data on bone density; and
- Allow faster, better, large-scale scoring of images for clinical trials, for example in osteoarthritis. Automated image analysis will eliminate variability among researchers.
A number of automated radiological image processing software applications, such as one for opportunistic bone density in CT scans, are under review by the U.S. Food & Drug Administration, says Dr. Hügle.
“I think that within two to three years, AI will become a reliable partner for rheumatologists in supporting image-based diagnosis,” Dr. Hügle says, adding that he thinks AI will “sneak in” to the daily lives of rheumatologists, and once it becomes familiar it will quickly become essential and normal.
Glossary of Terms3,6
Artificial Intelligence | Field of computer science since the 1950s, referring to the creation of computer systems to perform a task that typically requires human intelligence. |
Machine Learning | A subfield of AI since the 1980s in which a computer learns automatically from the data presented to it, mostly in a supervised learning situation. |
Deep Learning | A subset of machine learning since the 2010s that uses artificial neural networks with multiple layers to decode (imaging) raw data. |
Learning Curve
To help rheumatologists understand how AI and machine learning may be applied to imaging in rheumatology, Dr. Stoel outlines different ways these tools may be used to interpret digital images:3
- Fully human interpretation (mostly used in daily clinical practice): Images are interpreted by a clinician by first detecting abnormal structures in the image (i.e., detection) and subsequently determining what type of lesions they are (i.e., classification). In this approach, AI is used only to produce the images by reconstructing images from raw information from the scanner and by enhancing the appearance of the images;
- Hybrid or computer-aided approach: The computer helps the clinician detect suspected areas and/or classify lesions. Because computers (and in some cases clinicians) always need numerical measurements to perform classification, certain characteristics of the lesion need to be measured first. This intermediate step is called quantification. Usually these characteristic features are specified by a computer scientist in consultation with a clinician (e.g., shape, size, brightness; also known as hand-crafted features); and
- Fully automatic interpretation: here the whole process is automated fully. This can be done by still following the consecutive steps of the hybrid approach—automatic detection, quantification and classification—but hand-crafted features would still need to be specified by human researchers. This could be avoided by letting the computer learn discriminative features independently, without human interaction, by using machine-learning techniques, such as artificial neural networks.
When applying AI to imaging in rheumatology, Dr. Stoel explains that automation may be preferred and is particularly relevant for clinical trials due to reductions in cost, time and observer variability, as well as requiring less training and fewer skills.
He highlights ongoing research on automated image analysis methods to detect and assess the main musculoskeletal components of rheumatoid arthritis (RA)— synovitis, tenosynovitis and bone marrow edema, bone erosions and cartilage loss. Most research has gone into automatic quantification of cartilage loss, with early developments in AI using plain radiographs to quantify joint space in the hand and knee, and more recent AI methods to automatically measure joint spaces within the wrist.
Newer developments focus on using AI methods with magnetic resonance imaging scans to measure cartilage thickness and volume, and deep learning to detect knee cartilage and further classify cartilage lesions.
‘I think imaging is the entrance door for AI in rheumatology.’ —Thomas Hügle, MD, PhD
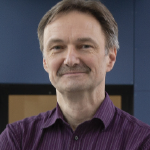
Dr. Stoel
For some situations, says Dr. Stoel, automation is necessary: for example, when the amount of imaging data is beyond what can be assessed manually or to detect subtle differences in brightness (i.e., intensity) not accessible to the human eye.
This last point—limits of the human eye to detect differences in imaging intensities—has spawned a separate field of AI research. Called comparative imaging, this area of AI is focused on providing the radiologist or rheumatologist with different images to help them find locations of—for example—progression or regression of joint disease associated with RA.
Examples of work being done in this area include software Dr. Stoel and his colleagues have developed that can show inflammatory changes in vertebral lesions in axial spondyloarthritis, as well as software developed to map local changes in lung density over time in patients with systemic sclerosis.
These are some of the basic ways in which AI can be used in rheumatology to enhance imaging interpretation. Dr. Stoel also mentioned limitations, such as the need for high-quality inputs to generate valid algorithms, the need for human expertise when developing the automated design for neural networks, the need for strict quality control of training, test and validation sets, and proper optimization of the imaging protocol.
Dr. Stoel thinks it unlikely that AI and machine learning will replace humans, but that a hybrid scenario, using both human and artificial intelligence, will be the most likely paradigm going forward.
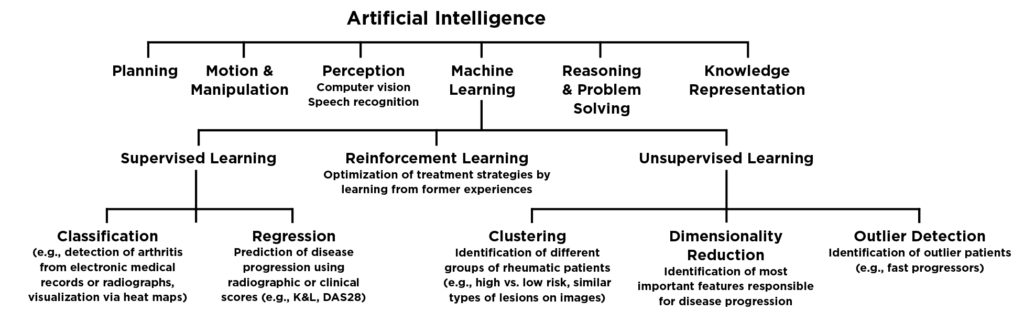
Figure 1. Created and copyright by Thomas Hügle; printed with permission
Change Is Coming
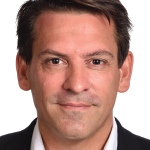
Dr. Hügle
In recognition of the importance of big data and such tools as AI and machine learning to the practice of rheumatology, the European League Against Rheumatism (EULAR) published recommendations to create a framework for how to apply big data in rheumatic and musculoskeletal diseases.5 According to EULAR, big data provides unprecedented opportunities that can deliver transformative discoveries in research and practice. The ultimate goal of using big data is to improve the health, lives and care of people, but ethical issues related to transparency, identity, confidentiality and privacy must be taken into consideration.
Prof. Laure Gossec, a rheumatologist at Sorbonne University, Paris, and the lead author of the EULAR recommendations, emphasizes that big data is coming. “It will change the way we perform studies, and also within a few years the way we diagnose and treat patients,” she says. “The methods are quite different from the usual analyses, and rheumatologists need to be aware of this big change coming up.”
As rheumatologists, like all clinicians, become more familiar with AI and machine learning and its capabilities, it is important to understand the benefits and limitations to ensure appropriate use of these sophisticated tools.
Dr. Hügle says AI will support and empower physicians. “There is much room for improvement in patient care in rheumatology,” he says. “If doctors have more time for interaction and difficult tasks and if a patient gets an automated second or third opinion, that’s a good thing.”
Mary Beth Nierengarten is a freelance medical journalist based in Minneapolis.
References
- Laghi A. Cautions about radiologic diagnosis of COVID-19 infection driven by artificial intelligence. The Lancet (Digital Health). 2020 May; 2(5):e225.
- Engler A. A guide to healthy skepticism of artificial intelligence and coronavirus. Brookings Institute. 2020 Apr 2.
- Stoel B. Use of artificial intelligence in imaging in rheumatology—current status and future perspectives. RMD Open. 2020;6:e001063.
- Hügle M, Omoumi P, van Laar JM, et al. Rheumatol Adv Pract. 2020;4(1).
- Gossec L, Kedra J, Servy H, et al. EULAR points to consider for the use of big data in rheumatic and musculoskeletal diseases. Ann Rheum Dis. 2020 Jan;79(1):69–76.
- Pesapane F, Codari M, Sardanelli F. Artificial intelligence in medical imaging: Threat or opportunity? Radiologists again at the forefront of innovation in medicine. Eur Radiol Exp. 2018 Oct 24;2(1):35.